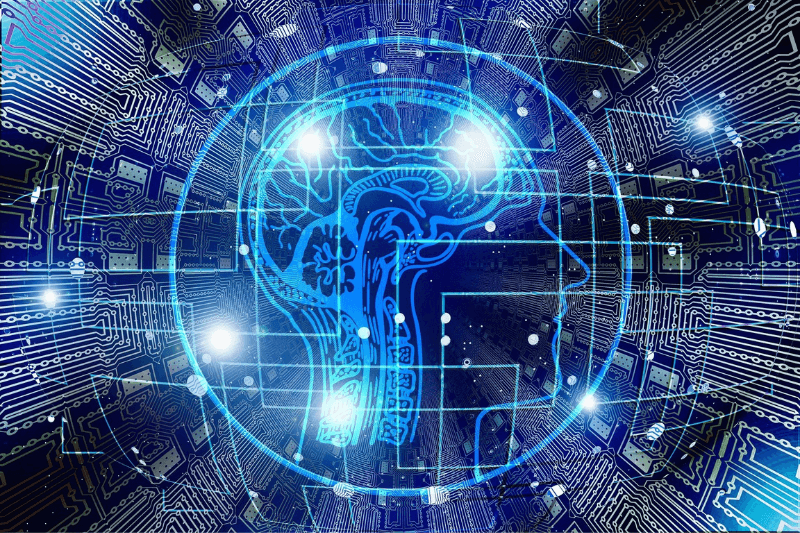
Deepfakes: Exploring Ways To Counter A Prominent Geopolitical Risk Of AI
One of the most concerning risks of artificial intelligence is its capacity to produce audio-visual content that depicts real individuals in fictitious situations – or deepfakes.
Manipulated videos can swing elections and erode public trust. They could even pose threats to financial markets by portraying key executives negatively to plummet a company’s stock price.
In the G20 Delhi Declaration, member countries highlighted their commitment to a pro-innovation approach in regulating AI, factoring in the risks posed by the technology.
Countering Deepfakes Through Detection And Provenance Techniques
In July this year, the G20 Conference on Crime and Security in the Age of NFTs, AI and the Metaverse specifically underscored the risk of malicious actors using deepfakes.
Moreover, deepfakes are known to have a significant social impact due to their ability to leave an imprint on people’s minds, even after being disproved.
Detection and provenance remain a couple of possible strategies to counter the damaging technology. The first one involves determining the authenticity of an image through algorithms.
However, the method isn’t rid of limitations. While detection methods usually look for visual inconsistencies, when content undergoes significant distortion, these indicators might vanish.
Keep Reading
Veins change colour when the heart pumps blood. Intel’s FakeCatcher collected data from blood flow signals all over a person’s face to identify manipulated content.
According to the BBC, the technology identified lip-synced deepfake videos well. But when the resolution of a video was substantially distorted, the detector was more likely to deem it as fake.
Deploying A Combination As Simply Banning Deepfakes Will Be Ineffective
The second method is provenance, which embeds media with metadata tracking attributes like authorship, creation data and edit history.
While provenance is known to reduce trust in deceptive content, it was found in cases where provenance data is incomplete, users might distrust even genuine content.
Therefore, deploying a combination of detection and provenance techniques could be useful as simply banning deepfakes will be ineffective due to the cross-border nature of transmission.